Exploring the Future of AI Scaling: Beyond the Constraints
Key Barriers to AI Scaling
AI's trajectory is marked by significant obstacles, primarily in four critical areas:
- Power consumption
- Chip manufacturing
- Data availability
- System latency
Revolutionizing Power Management
Power efficiency is a major concern for AI systems today. Innovations in energy-saving technologies and advanced power optimization systems are paving the way to reduce energy costs and carbon footprint.
"The best way to predict the future is to create it." — Peter Drucker
Advancements in Chip Manufacturing
The quest for smaller, faster, and more efficient chips remains a focus as it directly impacts AI's processing capabilities. Recent breakthroughs have improved chip architectures, aligning with the semiconductor industry's push for more innovation.
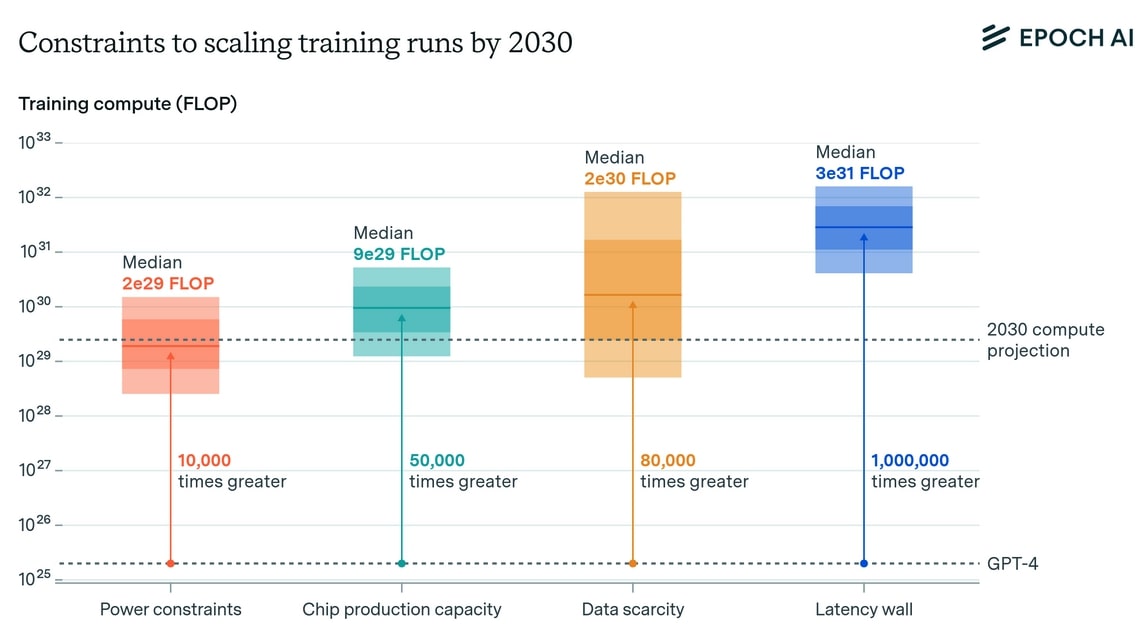
Data: the Fuel of AI
The need for vast amounts of data to train AI models is another critical issue. Partnerships with data-rich platforms and advanced data-processing algorithms are crucial for the future of AI scaling. Discover insights from leading tech analyses on advancing data use in AI.
Mitigating Latency
Latency affects real-time AI applications profoundly, from autonomous vehicles to instantaneous translation services. Cutting-edge techniques in networking and processing speeds are essential to overcome this barrier.
Check out expert discussions on LinkedIn about reducing latency and its impact on AI.
The Future Path Forward
While challenges remain, the strides being made today promise a future where AI is more scalable, efficient, and integrated into daily life. Watch this in-depth YouTube video where tech leaders discuss the horizon of AI scalability and innovation.
Moreover, keeping abreast of ongoing research and development is crucial for stakeholders in the AI arena. Explore related articles and stay informed through ResearchGate's latest publications on AI scaling challenges.