Unveiling the Perils of Overtraining: A Deep Dive into Large Language Models
The Double-Edged Sword of Extended Pre-Training
The quest for enhanced AI capabilities often leads organizations to train language models with ever-expanding datasets. However, recent insights indicate that excessive pre-training may lead to what researchers term 'catastrophic overtraining.' This phenomenon can diminish a model's ability to generalize and perform effectively across different contexts.
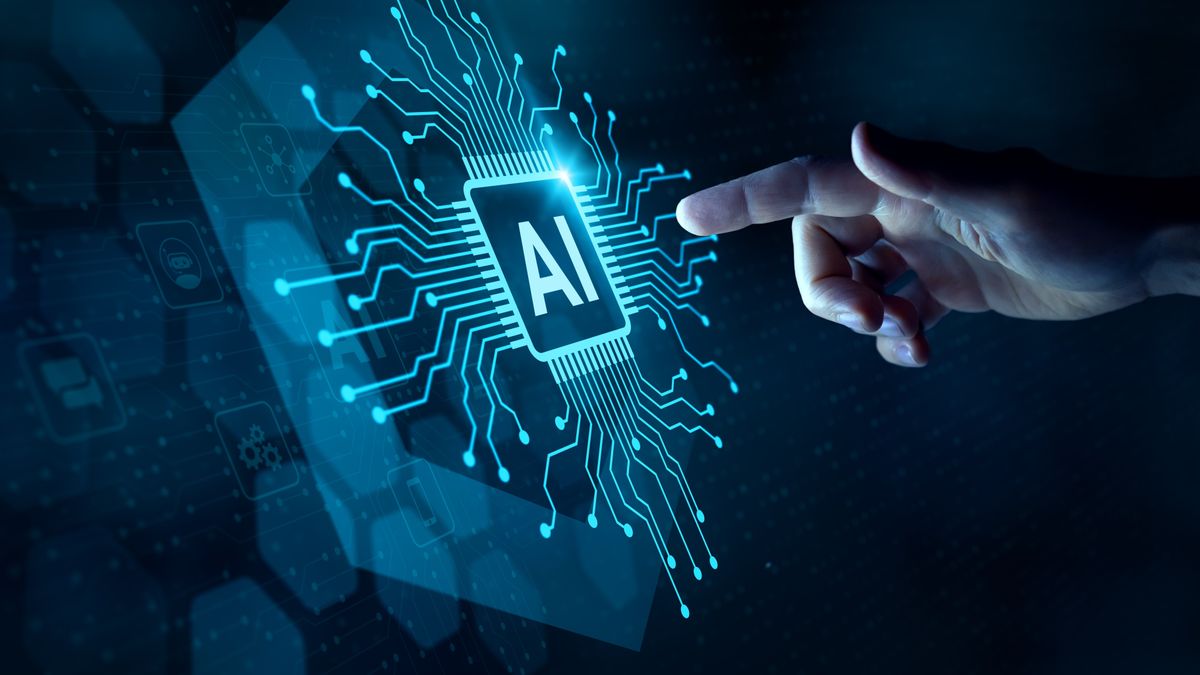
Insights from Top Researchers
Experts from top-tier universities, including MIT and Stanford, warn that extending pre-training might introduce diminishing returns. Thomas G. Dietterich, a renowned AI researcher, mentions,
"The key is to find the sweet spot where the data is just enough to maximize the model's performance without leading to over-reliance on specific patterns."Such insights underscore the fine balance between data volume and training quality. Read more about AI training methodologies.
Understanding Catastrophic Overtraining
- Pattern Overfitting: Too much data might cause a model to memorize rather than learn.
- Reduced Flexibility: Over-specialization could make LLMs less adaptable to new information.
- Resource Intensiveness: Increased pre-training demands substantial computational power, adding costs without assured returns.

The Road Ahead for AI Development
As the AI landscape evolves, it becomes paramount to balance ambition with practicality. Researchers propose integrating complementary efficiency-focused techniques with a moderate increase in data volume. This approach could potentiate sustainable AI growth, allowing for resource-efficient training without compromising quality. Explore related strategies in a LinkedIn article.
Implementing Efficient Educational Models
AI training doesn't exist in isolation—educational models applied to LLMs can be likened to efficient teaching methodologies. Using Amazon's educational resources, developers can learn to leverage AI in versatile and transformative ways. Find out more in the Wikipedia entry on educational technology.
Concluding Thoughts: A Call to Action
The AI industry stands at a critical juncture, with opportunities to shape future models that are both powerful and adaptable. By focusing on maintaining a balance between data size and model efficiency, developers can ensure AI remains a tool for innovation, rather than an overburdened system. Engage with ongoing discussions at the AI development forums.